In a perfect world, academic researchers would have the time and resources to order as many antibodies as possible and rigorously validate them to determine the best performing, most reliable product.
Today, this is not the case.
Scientific reliability expert C. Glenn Begley, MB, BS, PhD, chief scientific officer at TetraLogic Pharmaceuticals, knows more than most about this dilemma. In the early 2000s, scientists in the Hematology and Oncology Department at Amgen, led by Begley, found that 47 of 53 “landmark” preclinical studies were irreproducible (Begley and Ellis 2012). Antibodies were a major reason these studies failed. They are a key component in many biomedical studies and are used in fundamental techniques such as western blotting, immunoprecipitation, flow cytometry, and immunohistochemistry, but numerous studies conducted by other groups have demonstrated that many commercial antibodies are simply unreliable (Michel MC et al. 2009, Egelhofer TA et al. 2011, Berglund L et al. 2008). They bind to the wrong targets or to multiple targets.
Begley says large biopharmaceutical companies typically test many antibodies, sometimes going as far as generating their own. Conversely, many academic labs work with just one antibody, purchased from one of many commercial sources. The choice of antibody is often dictated by precedent: how many times have prior investigators cited that particular reagent? Even though on many occasions the cited publications failed to include critical controls.
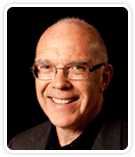
Chief Scientific Officer
TetraLogic Pharmaceuticals
Malvern, Pennsylvania, USA
In light of his newly published Nature editorial (Begley CG et al. 2015), coauthored with Alistair Buchan and Ulrich Dirnagl, we spoke to Begley about what’s driving antibody unreliability. Resource constraints are an obvious answer, but he believes the incentives within academia do just as much harm by preventing the adoption of validation techniques. As both a former professor and current head of a biotech R&D group, Begley says that academic researchers can apply a few best practices from industry that will improve the reliability of their research.
The Resource Struggle
Scientists in academia and industry think differently about validation. Large biotechs must scrutinize their results to ensure the data are robust and a potential product is safe. The downstream investment is many millions of dollars. Thus, they can economically justify investing heavily in antibodies and validation time.
Begley says that his scientific teams at Amgen would attempt to validate a wide range of antibody products in-house.
“(We) purchased antibodies from most of the manufacturers and then would pretty stringently seek to validate them before using them in any experiment,” Begley says. “We saw considerable lot-to-lot variations, so that validation was really necessary with every new batch of antibodies.”
Unfortunately, this level of validation and scrutiny is beyond the reach of the academic laboratories responsible for the bulk of our scientific knowledge. While academic researchers recognize the issue of reagent unreliability, most cannot afford to order multiple vials, nor can they afford the downtime involved in validating each new batch of reagents. Begley says academic scientists instead focus on optimization, rolling the dice on reproducibility.
“The fundamental difference is that in academia you take it for granted that an antibody does what it says it does. It’s unusual for a scientist in that setting to actually test the product to ensure that it works the way it was intended to work.”
“In (the biotech) industry, too much relies on that resource (antibody) and the decisions that are going to be made subsequently, so you have to check it thoroughly,” say Begley.
Underlying Incentives
For academic research to become more reliable, Begley and other industry leaders call for greater transparency, the use of positive and negative controls, and more validation data (Baker 2015). While some may see these steps as prohibitively expensive, there are deeper environmental pressures that oppose the calls for change.
Begley believes the issue of irreproducibility is intertwined with the ways academic scientists are currently incentivized. Success in academia is measured largely by a scientist’s publication record — the impact of the journals and the number of papers they can generate.
“That is how investigators are judged; that’s what determines whether or not they’ll be able to put bread on the table.”
Begley points out that journals such as Nature and Science are taking steps to address the issue, updating guidelines for experiment protocols and creating a checklist of best practices. There is also a push to begin reviewing primary data and analyzing validation techniques as a category of their own.
However, journals can do only so much when the culture within the laboratories and institutions emphasizes quantity over quality of research. Once scientists pass peer-review, it’s easy to move onto the next project. Begley says there is a lack of disincentives for researchers who don’t apply proper scientific rigor.
“You know, I’m quite cynical. In biological systems, almost whatever the results, they can be cast in a way that supports preconceived biases. If the results aren’t exactly what you want, it’s easy to ignore them, or to keep looking at the results until you find something that satisfies p < 0.05. There should be more focus on the research process rather than simply focusing on the result.”
A Middle Ground
Occupying a middle ground, small biotechs can provide a great deal of insight for academics keen on improving the reliability of their science. Like academic labs, early-stage commercial companies typically have limited resources. And like their larger biotech counterparts, they must be extremely rigorous with their science, as they share the pressures and long-term goals of larger biopharma companies.
“A small biotech is really not all that different from academia, in terms of the (resource) constraints,” says Begley. “They don’t have the resources to be able to do what a big company takes for granted.”
Despite this, Begley says early-stage companies are still held accountable for the validity of their work. The principle of “failing early and cheaply” holds true; if a drug candidate doesn’t stack up, biotechs of any size want to find out as early as possible, before larger investments are made. This drives a culture that challenges the data and findings to ensure the results are right.
As an industry consultant and chief technology officer of a small biotech, Begley advises early-stage companies on how to position their pipeline for acquisition. For the most accurate results, he stresses the need for positive and negative controls. Ideally many antibodies would be ordered and compared, to find the best performing product. However, Begley also notes that many small biotechs simply cannot afford to undertake this level of validation.
So how do small biotechs go about validation and what can academics learn from them?
Trial-size batches of antibodies are one option. These allow laboratories on a budget to sample different products, filtering out those that don’t work. Scientists take action, identifying poor-performing antibodies early in the research process.
“Certainly it would be useful to know that an antibody works before you buy a whole vial,” says Begley.
Another budget-conscious validation practice is to perform blinded experiments, to ensure researcher bias doesn’t contaminate interpretation of the results.
“It’s something really anyone can do and it’s extremely important,” says Begley. “The first question I ask when I see results from another company or academic group is, Were the experiments blinded?”
Outside of medical research and behavioral studies, blinded studies in life science fields are not the norm, according to researchers from Australian National University (Holman L et al. 2015). In biological research, they found only ten percent of all studies are blinded. Moreover, studies that weren’t blinded showed distinct markers of observer bias.
While many small biotechs accept blinded experiments as a best practice, academic labs are reluctant to assign multiple researchers to a single task. Even if this practice does cut down on errors, it doesn’t help academic researchers achieve success within the current research model. It can even impede their progress toward publication. According to Begley, academic scientists need to build a “perfect story” with results that all point to the same conclusion. Studies may be subconsciously angled toward certain results, or conflicting data dismissed. However, if there are two scientists working on one task they will be checking one another and self-censoring, so the data are more likely to represent the full — somewhat imperfect — scientific picture.
For Begley, this underscores the importance of performing blinded experiments as a way for scientists to self-censor. Without checks in place, scientists with the best intentions can subconsciously bias their results.
“It’s critically important to have checkpoints in place. We’re all human. We all see what we want to see.”
Finally, Begley believes that negative and positive controls cannot be swept aside, despite the expense and time required to perform each.
“They are hard to generate, but that doesn’t mean you shouldn’t do them. If you don’t have positive and negative controls it’s impossible to interpret the data.”
When it comes to experiments such as western blots, Begley believes more transparency is needed. Typically, only blots that “worked” are shown, with no indication of how many didn’t. Compounding this selectivity is the fact that once an experiment delivers the needed results, the investigator moves on, which means those results aren’t confirmed. For published results, many journals routinely accept cropped gels that highlight the band of interest and remove any other bands, which may signal a nonspecific antibody was used. Likewise, there is no size control shown to put the band into context and confirm for the reader that it’s the right molecular weight.
Tackling the Issue of Incentives
For academia to change, a cultural shift must take place in all corners of the scientific realm. Begley and his peers advocate for a multipronged approach that encourages journals, funding agencies, and institutions to support thorough research practices. There are a number of efforts underway that attempt to help academic groups deal with the real problem of resource allocation, and not-for-profit groups such as the Global Biological Standards Institute (GBSI) are attempting to tackle this head-on.
Beyond that, disincentives must be introduced to remove the academic emphasis on quantity over quality, and the “cherry-picking” of data to build a consistent science story. Some measures might include enforcing stricter penalties on scientists found falsifying results and demanding swift paper retractions when the science isn’t sound.
Within the laboratory, a critical component of science must be reintroduced — skepticism. Many do not challenge their own and others’ work, a practice that is widespread in industry laboratories and that keeps scientists accountable. Without this, it’s impossible to buy or generate antibodies that can deliver study reliability.
The researchers should not carry the whole burden of assessing the consistency of the products. It is also important that vendors do more to improve the performance and consistency of their antibodies, to give better starting points to researchers in academia and the industry.
For more information about the steps Bio-Rad is taking to address the antibody irreproducibility problem, read The Antibody Challenge: Bio-Rad’s Precise Solution, and read about Bio-Rad’s new PrecisionAb™ Antibodies validated for western blotting.
References
Baker M (2015). Reproducibility crisis: Blame it on the antibodies. Nature 521, 274–276.
Begley CG et al. (2015). Robust research: Institutions must do their part for reproducibility. Nature 525, 25–27.
Begley CG and Ellis LM (2012). Drug development: Raise standards for preclinical cancer research. Nature 483, 521–533.
Berglund L et al. (2008). A genecentric Human Protein Atlas for expression profiles based on antibodies. Mol Cell Proteomics 7, 2019–2027.
Egelhofer TA et al. (2011). An assessment of histone-modification antibody quality. Nat Struct Mol Biol 18, 91–93.
Holman L et al. (2015). Evidence of experimental bias in the life sciences: Why we need blind data recording. PLoS Biol 13, e1002190.
Michel MC et al. (2009). How reliable are G-protein-coupled receptor antibodies? Naunyn Schmiedebergs Arch Pharmacol 379, 385–388.